Understanding Annotation in Machine Learning
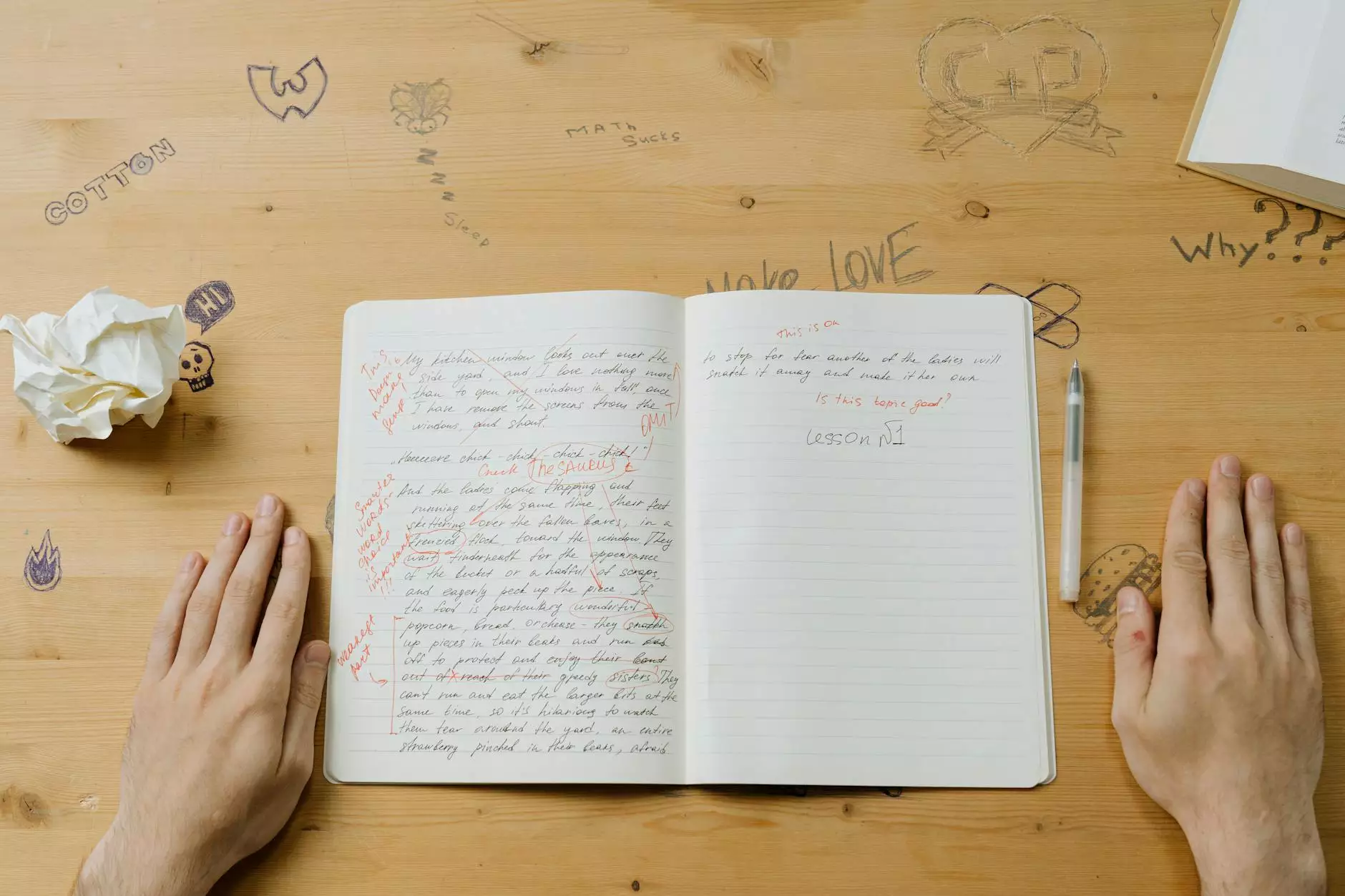
Annotation in machine learning is a critical process that involves labeling data to train algorithms effectively. This process is essential not just for accuracy but also for the efficacy of software development across various industries. Companies like KeyMakr have pioneered in providing annotation solutions that enhance machine learning models, driving innovation and efficiency in software solutions. In this article, we will delve deep into the nuances of annotation and its profound impact on businesses.
What is Annotation in Machine Learning?
Annotation refers to the act of adding notes or explanations to data, essentially allowing machines to understand and learn from that data. Machine learning relies on vast amounts of data, and without proper annotation, the algorithms might misinterpret the data, leading to inaccurate predictions. Here are some types of annotation:
- Text Annotation: Labeling text data such as identifying sentiments or classifying topics.
- Image Annotation: Marking objects within images, used extensively in computer vision.
- Audio Annotation: Adding labels to sound clips for tasks like speech recognition.
- Video Annotation: Labeling objects in video frames for real-time analysis.
The Role of Annotation in Machine Learning Algorithms
Annotations serve as a foundation for training machine learning algorithms. The quality and accuracy of the annotations directly influence the model's performance. High-quality annotated datasets lead to models that can generalize better to unseen data, ultimately enhancing the software development lifecycle.
1. Enhancing Predictive Accuracy
When data is accurately labeled, it significantly enhances the predictive accuracy of machine learning models. For instance, in software development, an annotated dataset can help in building models that predict user behavior more accurately, allowing businesses to tailor their offerings and improve customer satisfaction.
2. Improving Model Training
Annotation ensures that the training dataset is reflective of the real-world scenarios the model will face. This is crucial for creating robust software applications that need to perform reliably under various conditions. Quality annotations feed the algorithms the right information, thereby improving the overall training process.
3. Facilitating Complex Tasks
With the growing complexity of tasks handled by machine learning, annotation becomes even more crucial. For example, automatic driving software requires precise image annotations to identify pedestrians, road signs, and other vehicles. In this sense, annotation lays down the groundwork for systems that can perform complicated functions automatically.
The Business Impact of Machine Learning Annotation
Incorporating effective annotation practices in machine learning has far-reaching implications for business success. Here’s how:
1. Operational Efficiency
By leveraging machine learning models that are well-trained through proper annotation, businesses can achieve operational efficiency. Automated processes can handle repetitive tasks, freeing up human resources for more critical roles. This efficiency translates into cost savings and improved productivity.
2. Enhanced Decision Making
With access to better predictive analytics driven by annotated data, businesses can make informed decisions. This leads to strategic advantages in planning, inventory management, personalized marketing, and more. In the software development arena, this could mean launching products that align perfectly with market demand.
3. Competitive Advantage
In today's fast-paced business environment, staying ahead of competitors requires agility and data-driven insights. Companies that invest in quality annotation services can swiftly adapt their software solutions to evolving customer needs, ensuring they maintain a competitive edge.
Choosing the Right Annotation Service
Selecting the right annotation service is crucial for leveraging machine learning effectively. Here are some factors to consider:
- Expertise: Choose a service with a proven track record in your industry.
- Quality Assurance: Ensure they have a robust QA process to maintain accuracy in annotations.
- Scalability: The service should be scalable to meet the growing demands of your business.
- Technological Integration: The capability to integrate seamlessly with your existing systems is paramount.
Best Practices for Effective Annotation
To achieve the best results from annotation in machine learning, best practices should be followed:
1. Define Clear Guidelines
Establish clear guidelines for annotators to follow. This reduces inconsistencies in the data labeling process and ensures that everyone is aligned on expectations.
2. Use Quality Control Mechanisms
Incorporate quality checks and measures. Regular audits of labeled data will help in identifying and rectifying errors quickly.
3. Engage Experts
For certain tasks, especially in specialized fields like healthcare or law, it is crucial to involve domain experts in the annotation process. Their insights ensure that the data is labeled accurately and meaningfully.
4. Leverage Technology
Utilize AI-powered tools for annotation where possible. These tools can speed up the process and maintain consistency, though human oversight is still invaluable for quality assurance.
Future Trends in Machine Learning Annotation
The field of annotation is evolving, and staying updated on future trends is essential for businesses looking to implement machine learning effectively.
1. Automated Annotation
With advancements in AI, automated annotation is becoming a more viable option. While it may not completely replace human annotators, it can significantly reduce the workload and improve efficiency.
2. Real-Time Annotation
As businesses look for faster insights, real-time annotation capabilities will become increasingly important. This will allow for instantaneous data labeling and analysis, leading to quicker decision-making.
3. Enhanced Collaboration Tools
Future annotation services will likely focus on improving collaboration between teams. Tools that allow for seamless communication and feedback loops will enhance the annotation process, making it more efficient.
Conclusion
In conclusion, the importance of annotation in machine learning cannot be overstated. It is an integral part of the software development landscape that impacts business outcomes significantly. As businesses continue to harness the power of machine learning, ensuring high-quality annotations will be key to unlocking their full potential. By understanding the nuances of annotation and following best practices, companies can position themselves to thrive in an increasingly data-driven world.
Embracing advanced annotation techniques is not just a choice; it's a necessity for businesses looking to stay ahead in innovation and efficiency. Emphasizing quality and accuracy in data labeling will lead to more reliable machine learning models, driving growth and success in your software development endeavors.
annotation machine learning